Our technology
Modern discovery efforts in antibody, TCR, vaccines, and cell therapies demand a robust foundation in data processing. Accurate and reliable annotation tools are fundamental for unlocking the full potential of immune repertoires and highly diverse synthetic libraries. With the advent of high-throughput sequencing, scalability has become paramount to fully explore the vast diversity within these datasets.
Annotated data must then be stored in high-performance databases that enable rapid retrieval and effortless access to large-scale datasets—a capability that becomes even more critical with the integration of emerging machine learning technologies. The ENPICOM Platform is meticulously engineered to meet these critical demands, ensuring that your discovery efforts are built on the strongest possible technological foundation.
Join the future of clone annotation
Experience and accuracy in clone annotation
Our clonal annotation tool, IGX-Profile, is the result of years of expertise in repertoire sequencing data annotation. The ENPICOM team has carefully optimized it to handle every step of the process with unmatched accuracy. From read-pair merging and quality filtering to UMI processing and chain-pair linking via barcodes, IGX-Profile annotates BCR, TCR clones, and other molecules with exceptional accuracy. The sensitivity of these annotations has been rigorously benchmarked against spike-ins across diverse datasets, demonstrating its reliability and effectiveness.
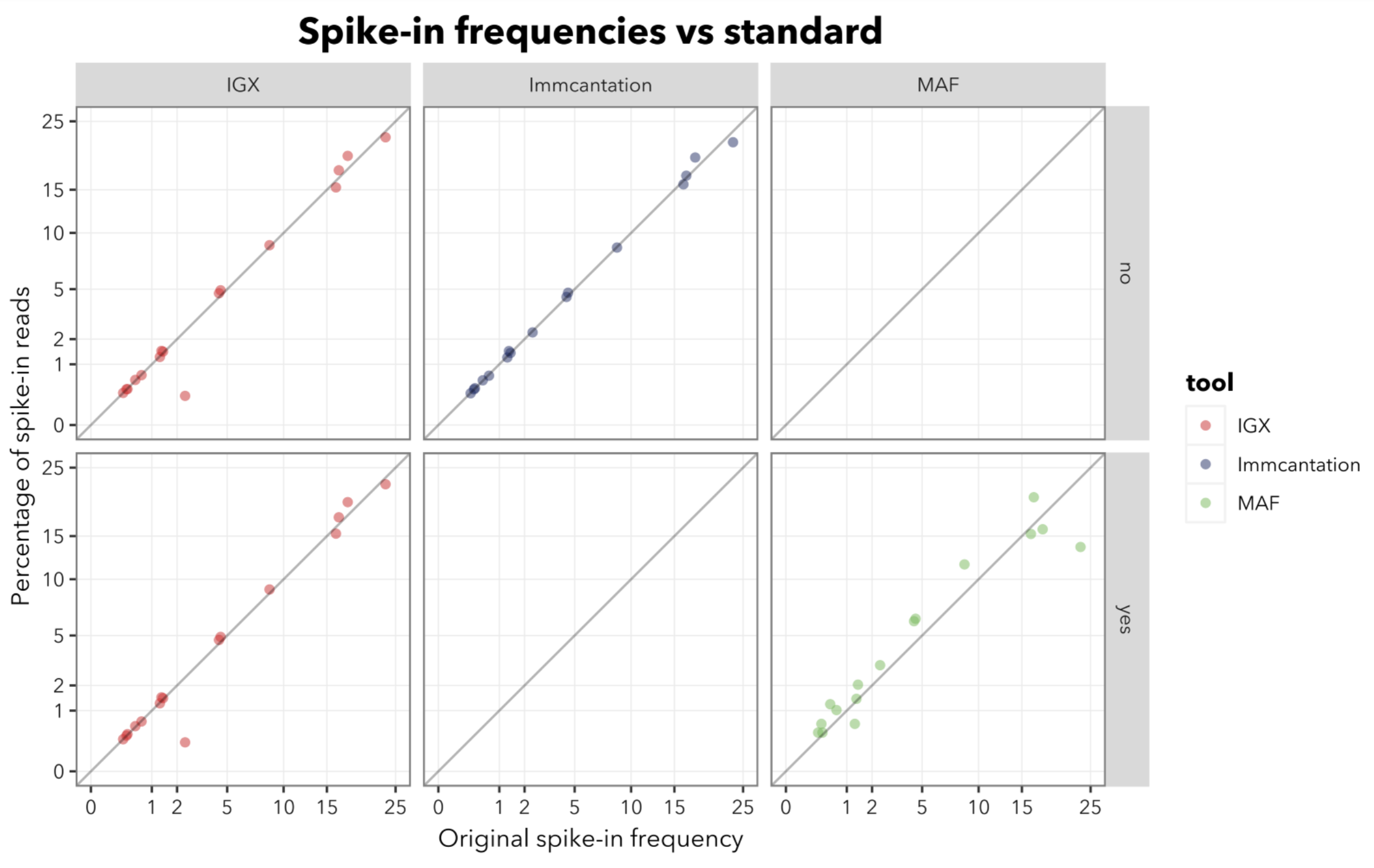
Blazing fast performance, built for scale
Purpose-built to handle datasets of any size, IGX-Profile delivers blazing fast performance. Whether handling Sanger Ab1 files or large-scale NovaSeq FASTQs, IGX-Profile enables seamless annotation and integration of all data within a unified platform. Capable of processing thousands of samples in parallel at speeds exceeding 1 million reads per minute, it effortlessly annotates billions of reads within just hours. This makes IGX-Profile the fastest annotation tool available, boasting ten times the processing speed of other public solutions, and significantly reducing both the waiting time and costs associated with large sequencing datasets.
Fine control with ease of use
Even with its powerful performance, IGX-Profile does not compromise on flexibility and user control. It offers extensive options for any amplicon/read configuration and quality control filtering through an intuitive interface. Whether processing single-chain VHH, scFv, or short-read 10x Genomics data, the configuration is straightforward with a simple drag-and-drop template. Scientists can also readily filter the data by read quality, sequence count, or UMI, restrict clones to those with both chains, and much more within a QC template. These templates streamline the setup process, ensuring consistency with SOPs and enhancing reproducibility across your projects.
Seamless data management for comprehensive discovery
Effective data management is crucial to discovery analyses including diverse experimental metadata and/or large sequencing datasets, and the ENPICOM Platform excels in this domain. All annotated data is stored in a rapid, scalable database that ensures effortless access and extraction for analyses. This database houses sequencing data and allows for the seamless association of any assay or in-silico generated data with individual clones, allowing you to integrate these in your analysis to find the best leads.
At the core of this system is Amazon’s Redshift Massive Parallel Processing (MPP) architecture, combined with our proprietary high-throughput SQL engine. This powerful combination allows for blazing-fast queries, enabling you to search receptor motifs among hundreds of millions in mere seconds. The database is built to scale to petabyte-sized datasets, accommodating all your historical data while seamlessly integrating new data from instruments like NovaSeq.
Foundation for machine learning
Beyond data storage, this environment provides an ideal foundation for training machine learning models. With its organized, scalable structure and rapid data retrieval capabilities, the platform ensures that your ML efforts are backed by the most comprehensive and efficient data management possible.
Industry leading security
Security of course is a top priority in our platform. We leverage AWS enterprise security, regularly conducting vulnerability scanning, penetration testing, and enforcing a zero-trust policy for database access. This ensures that your data is not only accessible but also protected to the highest standards.
- Security protocols
- ISO27001 compliance
- SSO integration
- 2-factor authentication (2FA)
Trusted by industry leaders
Transform your research with seamless data integration
and future-ready data science
Contact us for a demo